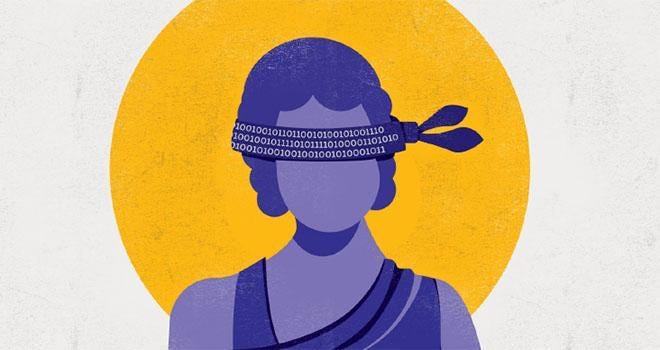
Image Source: Virtual Speech
Artificial Intelligence is a huge aid for mankind. We now have AI that can:
Help financial institutions assess the loan risks and credit worthiness before committing to bad loans
Find the right candidates for jobs thus helping companies save millions of dollars in talent acquisition
Analyse thousands of data points to develop patterns in human conditions and diagnose illnesses
and the list only goes on.
Picture this
You are a black woman who is a very successful account executive
Recently, you applied to a job that looked right up your alley but never heard back
Later, you’re surprised on hearing that a white, male acquaintance with a very similar career trajectory to yours was hired for that job
The recruiter then tells you that there could not have been a mistake, that they run an AI-based matching software. Apparently, your profile came out as a low match
This is a clear illustration of potential gender/racial bias in the AI algorithm.
How can a neural network possibly be biased?
An algorithm is as good as the data we train it with.
If the data is a direct reflection of reality, it will show women being hired less than men in a traditionally male-dominated industry like finance
The AI will pick up gender as a ‘label’ that affects hiring
The next time, you feed a woman’s profile to the algorithm, the AI will decide that since the gender is female, the chances of recruitment should go down
Some examples
Loans to black and hispanic people can be deemed more ‘risky’ and their interest rates can turn out to be higher.
People with traditionally muslim names can be surpassed by algorithms during application screening.
These are instances where the data inherently contains bias that the neural networks can pick up.
Bias in data selection
An algorithm for facial detection in mobiles was proven to work well only for white men.
Most black criminals have been wrongly tagged by the US COMPAS algorithm as people who might repeat committing crime in the future.
These are examples of selection bias in data that highlights one geography/ethnic group causing the algorithm to inflate results for that group.
Dangers of bias in AI
Scalability: The most apparent advantage of AI is that one neural network makes decisions that a thousand humans probably can together. On the other hand, we are scaling the human biases by that extent as well.
Fatality: These biases perpetuating in legal and healthcare systems can be life-threatening for people belonging to marginalised communities.
Irreversibility: If unchecked, widespread use of unchecked AI can cause extreme damage to the underrepresented and marginalized communities.
Potential Solutions
Pre-define equal opportunity outcomes: Specify clearly what the AI should accomplish for different diverse and under-represented/ oppressed groups in the society before starting to build it.
Choose data points consciously: Based on the diversity outcomes defined, choose data points from the entirety of the data to help cater to determined outcomes.
Determine checks and measures: You are bound to miss out on a few things in the initial definition. Define the metrics to keep a hold on the biases.
Continual tracking: Bias might always crawl back in again when a new data point is introduced in the environment. Keep checking for bias anytime a change is made to the algorithm or problem statement.
Make space for improvements: You will keep discovering new target groups that might be on the receiving end of the bias. Make space and enable the flexibility to keep adding them to your tracker.
Have a diverse team: Finally, you will not be able to get that diverse point of view alone. Incorporate a diverse team of people who bring in a unique point of view to the table.